Ongoing Projects
- Correcting numerical errors in Navier-Stokes simulations and PDE-solvers via Deep Learning
- PhiFlow: Learning long-term interactions via differentiable physics-solvers.
- Deep learning for particle-based (Lagrangian) simulations with Continuous Convolutions, e.g. for SPH
- Learning Similarity Metrics for Numerical Simulations
- CNN-patches: We compute flow descriptors based on flow invariants, which we use these to look up pre-computed patches of 4D data.
- ML-FLIP: This data-driven model captures sub-grid scale formation of droplets for liquid simulations.
- tempoGAN: Our GAN approach directly synthesizes a temporally coherent state of an advected quantity, such as smoke.
- Latent-space physics: This work focuses on pressure fields over time. In contrast to the others, it predicts the temporal evolution using the latent-space of a trained encoder network.
- Neural Liquid Drop: this method captures full solutions for classes of liquid problems in terms of space-time deformations, allowing for real-time interactions.
- Predictions of Reynolds-Averaged Navier-Stokes Flows around airfoils with a simple PDE-solving U-net architecture
Physics-Based Deep Learning book (PBDL): Our research efforts are summarized in this online Jupyter book. It contains an introduction of everything related to deep learning in the context of physical simulations. As much as possible, all topics come with hands-on code examples in the form of Jupyter notebooks to quickly get started. Beyond standard supervised learning from data, it looks at physical loss constraints, more tightly coupled learning algorithms with differentiable simulations, training algorithms tailored to physics problems, as well as reinforcement learning and uncertainty modeling. These methods have a huge potential to fundamentally change what computer simulations can achieve.
PhiFlow: A focus of our research and development efforts is our fully differentiable physics-solving framework PhiFlow. Having all functionality of, e.g., a fluid simulation running in TensorFlow opens up the possibility of back-propagating gradients through the simulation as well as running the simulation on GPUs. The framework support for a variety of differentiable simulation types, from Burgers over Navier-Stokes to the Schrödinger equation.
MantaFlow: Many of our research projects are based on a common codebase, the mantaflow solver. This solver is an open-source framework targeted at fluid simulation research in Computer Graphics. It has a parallelized C++ solver core, a high-level python API for defining scenes and quickly adapting the solvers. It is tailored towards quickly prototyping and testing new algorithms. Recently, we’ve also added tools and plugins to interface with the tensorflow deep learning framework. The long term goal is to build a flexible platform for machine learning projects involving convolutional neural networks and fluid flow. Below, you can find an introduction to get started with manta & tensor-flow, and more detailed tutorials will follow soon.
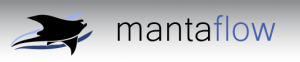
Just in case you haven’t found it yet, the official mantaflow homepage is this one: http://mantaflow.com
SpaTe: Currently, an important focus of our group is the ERC Consolidator Grant SpaTe. It aims for a fusion of space-time physics with machine learning algorithms that will allow us to fundamentally improve the way we work with computer simulations, and benefit forward as well as inverse problem solvers with physical constraints.
Details: https://ge.in.tum.de/research/research-spate/
realFlow: Previously, our group worked on the ERC Starting Grant realFlow. This grant, with a was aimed at novel simulation and reconstruction algorithms for fluid flows. The full title is “realFlow – Virtualization of Real Flows for Animation and Simulation” (StG-2015-637014). It’s goal was to improve the simulation of physical processes and, above all, make it possible to generate such simulations more quickly and realistically. A central component of this research are data-driven methods, and especially machine learning techniques with deep neural networks.
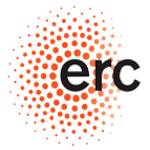
Details: https://www.in.tum.de/en/cg/research/ercstg-realflow/