Our paper “Scale-invariant Learning by Physics Inversion” was accepted at NeurIPS and is available now at https://arxiv.org/abs/2109.15048.
The key insight is that custom inverse solvers (SIPs) provide a powerful tool that outperforms differentiable programming and supervised training, here’s a preview, x^* the reference:
Even though we only use them for the physics part, SIP-training helps to reach levels of accuracy that are unattainable with other learning methods (the NN is still trained via simple backprop). The main takeaway is: consider replacing your regular backprop gradients through the solver with something better (e.g. an inverse)! The source code is available at: https://github.com/tum-pbs/SIP
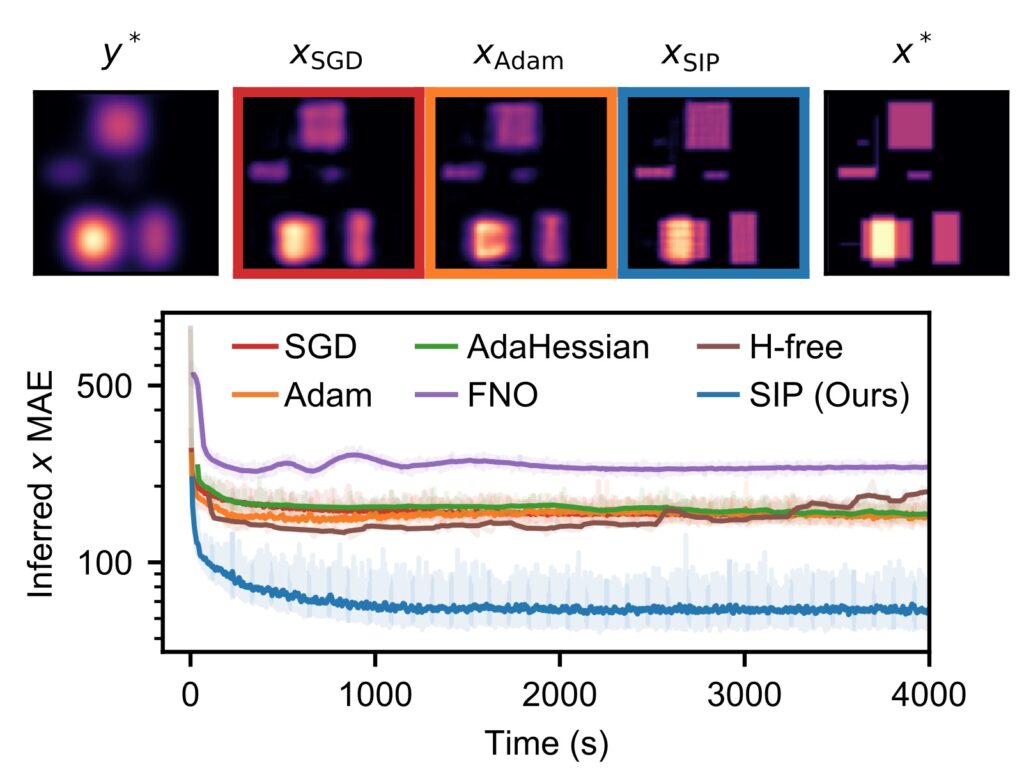
Full paper abstract: Solving inverse problems, such as parameter estimation and optimal control, is a vital part of science. Many experiments repeatedly collect data and rely on machine learning algorithms to quickly infer solutions to the associated inverse problems. We find that state-of-the-art training techniques are not well-suited to many problems that involve physical processes. The highly nonlinear behavior, common in physical processes, results in strongly varying gradients that lead first-order optimizers like SGD or Adam to compute suboptimal optimization directions. We propose a novel hybrid training approach that combines higher-order optimization methods with machine learning techniques. We take updates from a scale-invariant inverse problem solver and embed them into the gradient-descent-based learning pipeline, replacing the regular gradient of the physical process. We demonstrate the capabilities of our method on a variety of canonical physical systems, showing that it yields significant improvements on a wide range of optimization and learning problems.