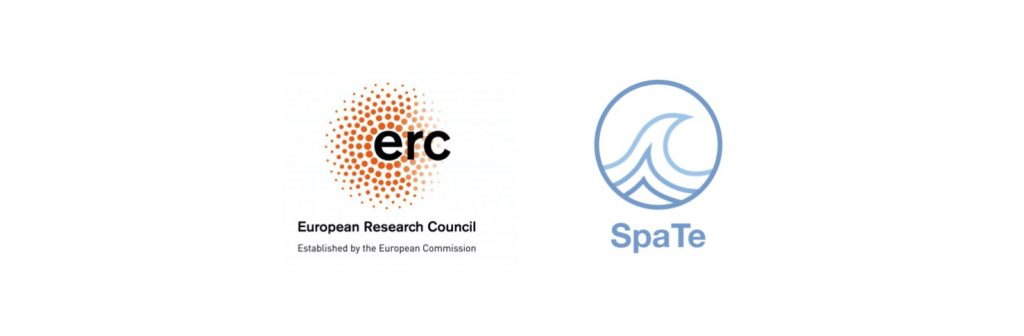
Principal Investigator: Prof. N. Thuerey
Overview: This grant project aims for a fusion of space-time physics with machine learning algorithms that will allow us to fundamentally improve the way we work with computer simulations, and benefit forward as well as inverse problem solvers with physical constraints. Hence, the central goal of this project – SpaTe – is to develop new classes of data-driven spatio-temporal methods and demonstrate their potential to drive the next generation of numerical simulations and computer animations.
It consists of three parallel and synergetic lines of work:
- Reduced Temporal Representations: Target learning generic and re-usable representations based on convolutional neural networks (CNNs) that target Eulerian space-time functions of physical problems.
- Physics-based Inverse Problems: Leverage advanced numerical techniques for discretizing the differential operators of model equations to arrive at robust, unsupervised learning algorithms for physical phenomena.
- Physical Understanding of Unstructured Data: Develop adaptive algorithms for sparse, Lagrangian space-time functions to analyze and disambiguate com- plex data sets such as point clouds without correspondences.
Project publications: