Our work on transonic flows over aerofoils is finally online: https://arxiv.org/abs/2403.17131
Apart from being accurate & stable. We also realized the trained models allow for efficiently performing a “classic” global instability analysis.
It was great to see the networks “really” learn the fundamental physics: When applied to a previously unseen mean flow, the eigenvalue spectrum of the trained network reliably captures the key modes that we’d expect from a transient case.
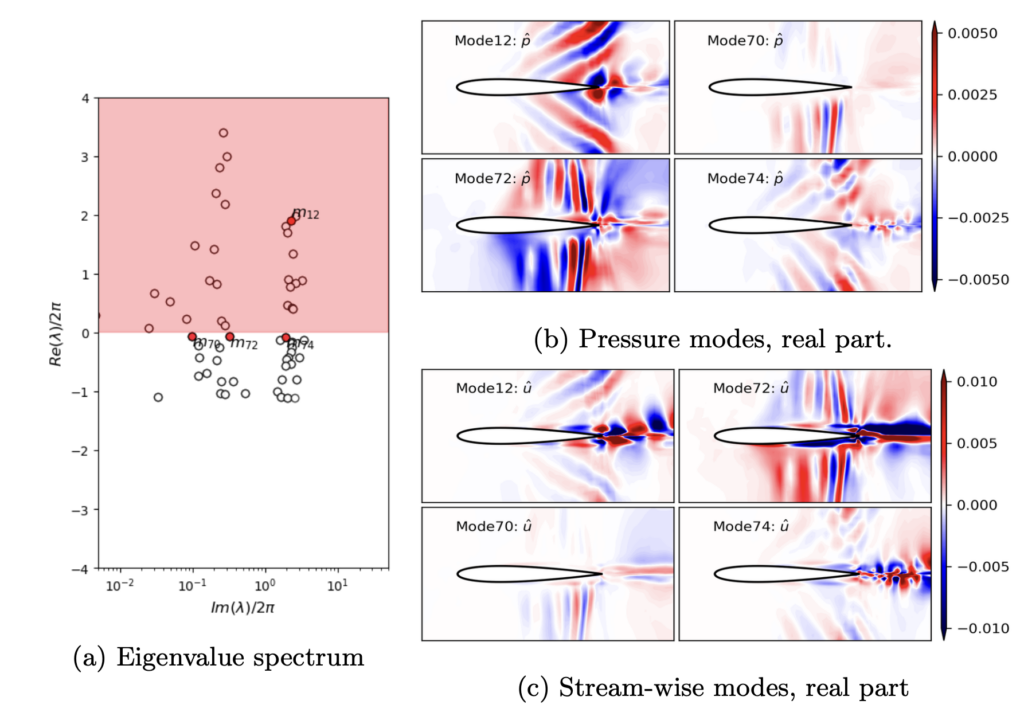
The unstable modes near 10^-1 along y (with positive real part) indicate the onset of buffet, i.e. growing large scale instabilities in the flow. The smaller modes around 2*10^0 are typical vortex shedding frequencies from the Kelvin-Helmholtz instability.
Full paper abstract: Effectively predicting transonic unsteady flow over an aerofoil poses inherent challenges. In this study, we harness the power of deep neural network (DNN) models using the attention U-Net architecture. Through efficient training of these models, we achieve the capability to capture the complexities of transonic and unsteady flow dynamics at high resolution, even when faced with previously unseen conditions. We demonstrate that by leveraging the differentiability inherent in neural network representations, our approach provides a framework for assessing fundamental physical properties via global instability analysis. This integration bridges deep neural network models and traditional modal analysis, offering valuable insights into transonic flow dynamics and enhancing the interpretability of neural network models in flowfield diagnostics.