We’d like to highlight a first one of our this year’s ICLR papers: “Diffusion Graph Nets” (Congrats to Mario 👏 ). The paper targets predicting complex distributions of flow states on unstructured meshes (https://openreview.net/forum?id=uKZdlihDDn). It’s not only highly efficient, but also excels at “completing” distributions: it works even if the training data contains only a fraction of the flow statistics per case. Above are some examples for turbulent flows in 3D over a wing.
It’s also worth mentioning that the code is already online at https://github.com/tum-pbs/dgn4cfd , complete with notebooks, flow matching, and the full hierarchical diffusion graph net architecture. If you’re trying it, please let us know how it works for you!
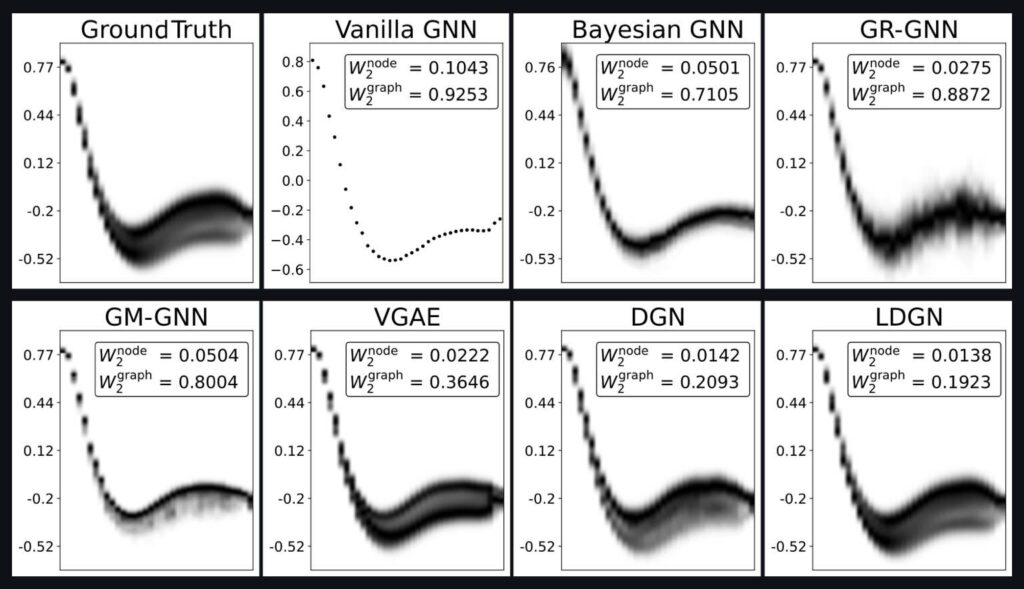
Full abstract: Physical systems with complex unsteady dynamics, such as fluid flows, are often poorly represented by a single mean solution. For many practical applications, it is crucial to access the full distribution of possible states, from which relevant statistics (e.g., RMS and two-point correlations) can be derived. Here, we propose a graph-based latent diffusion model that enables direct sampling of states from their equilibrium distribution, given a mesh discretization of the system and its physical parameters. This allows for the efficient computation of flow statistics without running long and expensive numerical simulations. The graph-based structure enables operations on unstructured meshes, which is critical for representing complex geometries with spatially localized high gradients, while latent-space diffusion modeling with a multi-scale GNN allows for efficient learning and inference of entire distributions of solutions. A key finding of our work is that the proposed networks can accurately learn full distributions even when trained on incomplete data from relatively short simulations. We apply this method to a range of fluid dynamics tasks, such as predicting pressure distributions on 3D wing models in turbulent flow, demonstrating both accuracy and computational efficiency in challenging scenarios. The ability to directly sample accurate solutions, and capturing their diversity from short ground-truth simulations, is highly promising for complex scientific modeling tasks.